Problem
The primary problems of aviation remain efficiency and safety, with deep complexities residing in the interplay and balancing of the two. The common understanding is that there is tradeoff between efficiency and safety, especially in the real world operations, as aircraft encounter weather and traffic, and operators simultaneously prioritize on-time performance and passenger comfort.
Additionally, the global push for sustainability has meant that decarbonization has become the intrinsic goal of efficiency, as aviation produced 2% of total global emissions in 2022, while pledging carbon neutrality by 2050.
Safety
Turbulence is the leading cause of airline safety incidents, accounting for 37% of all recorded incidents in the United States (this is a broad measure that includes ground, cabin and runway incidents). Traditional methods of navigating around turbulence are largely dependent on pilot’s intuition, backed up by training and accumulated experience.
In this way, pilots and operations teams are forced to contend with a complex decision-making process, while only having a coarse understanding of where turbulence may or may not be.
Ultimately, the limiting factor is the current level of technology involved with turbulence prediction. The granularity of current systems is 100x100km, and are primarily based on vertical wind shear (one of many factors in the manifestation of turbulence). This creates a gap between what is detectable by current systems, and what is relevant to pilots.
Currently, the best supplementary tool is PIREPS (Pilot Reports), that are circulated only after an initial aircraft has encountered turbulence significant enough to manually report. Apart from being retroactive, the extent and spread of turbulence remains unknown. PIREPS prompt wide, inefficient diversions by the following planes, often without the guarantee of complete avoidance.
This is an intractable problem that airlines are continually challenged by, and have been looking for solutions as far back as we can find.
The problem is also set to get worse, with academic studies finding that increased energy in the atmosphere due to global warming causes an increase in the incidence of turbulence. Conventional methods to avoid turbulence are expected to lose effectiveness.
Turbulence is already the leading cause of safety incidents, and set to get worse
Efficiency
Fuel efficiency is intrinsically tied to decarbonization, however balancing overall operational efficiency, through traffic, passenger comfort, safety and on time performance, requires increasing fuel consumption. Between 20-30% of airline expenditure is fuel. With fuel prices rising, ticket prices are increasing to compensate, putting pressure on affordability.
Isolating and solving a single problem may only produce limited benefits, may be impossible to act on due to constraints or conditions, and may be factored out when unintended costs are considered. This is because the complexities of aviation are so tightly coupled with each other.
Solution
Untangle
We apply Machine Learning and Artificial Intelligence to untangle the complexities of aviation on behalf of pilots and operations teams. Our approach uses software to reduce fuel consumption and carbon by ingesting, reprocessing and upgrading flight plans with comprehensive, multi-objective, strategic flight optimizations for pilots, resulting in increased operational resilience.
The immediate effect is finding 4-5% (average maximum) more fuel optimization during cruise without hardware changes, regardless of fleet age, regardless of current or future engines and fuels.
What makes it different?
We take a fundamentally different approach to producing optimizations compared to conventional flight planning, and even compared to contemporary third party solutions. Untangle is built to solve multiple problems simultaneously with fine granularity, then comprehensively balance the results based on the needs of the pilot, and the goals of the operations team.
Comprehensive and balanced
In this way, Untangle is a framework for several ML/AI models to work in modular fashion. Each model is developed, tested, and validated independently, using state of the art techniques. The current version of Untangle combines a more accurate fuel model, a precise altitude optimization, and a high resolution global turbulence forecast. By themselves, multiple new, cutting edge models would facilitate new levels of optimization. But these benefits would remain fragmented, each siloed in its own domain. Together, with Untangle orchestrating them, they represent a method to simultaneously increase safety and efficiency.
Upgradable and expandable
Although the performance has been validated for all current models, none of them are standing still. Each is continually refined, and continuously developed based on what is learned. New versions, with better performance are on the way. Similarly, the current features represent the start of Untangle’s roadmap. More models are in the works, and new features will be added.
Untangle itself, as a framework for synthesizing and balancing these objectives, is continually improving, adding to the ways optimizations can be tailored to each carrier, and each pilot.
Multi-objective and tailored optimization
By simultaneously computing multiple objectives for in-flight operations Untangle is able to treat each as a parameter. The differing priorities and brand values of different airlines can be expressed by setting a desired balance between safety, comfort, on-time performance, and fuel efficiency.
For Operations
The tailoring of optimizations enables operations teams to set goals for the year at the organizational level, and set parameters for how flights will be optimized in order to realize those goals. These parameters can be tweaked at any point throughout the year, and apply to all flights that get optimized. Hitting operational goals should produce a knock-on effect of freeing up capital to devote to the purchase of SAF or SAFc, further accelerating decarbonization efforts.
For Pilots
Within this operational tailoring of optimizations, pilots still have the freedom to choose the best option to fit the context. Our technology is built to increase situational awareness through higher resolution forecasts, and precise avoidance. Rather than remove agency from pilots, or insert tactical instructions, we aim to provide strategic options. Multiple optimizations are generated for each flight, each optimization representing a strategic priority: safest, fastest, or most fuel efficient. The aim is to supplement the experience and skill of the pilot, and reduce the cognitive load involved in complex in-flight decision making.
Current features: upgrading fundamentals
Feature 00
Turbulence prediction
Our turbulence prediction capabilities come from building a physics based machine learning model developed to address a specific pain point for aviation: turbulence is a largely unseen and safety problem, that burns a significant amount of extra fuel. What if we could have a high resolution map of turbulence, and precisely avoid it? In an industry first, the turbulence prediction model does just that, with preliminary validation showing an increased prediction rate of 18x over VWS.
Conventional wind shear model
NABLA Mobility turbulence prediction
Launch capabilities include:
60 hours of prediction, minimum
Predictions that include CAT and MWT
High resolution data (0.25 degrees)
Global coverage
FL140 to FL450
18x improved accuracy vs VWS models
18x
enroute detection success
Feature 01
Altitude optimization based turbulence avoidance
The altitude optimization model takes advantage of the high resolution turbulence prediction as a base by looking at conditions between waypoints, at an interval of 15NM, rather than only at waypoints, fundamentally opening up the options available with a fine grain grid. When it compares this high resolution reading of weather, it includes a granular understanding of the wind profile as well, in order to find tail winds and avoid headwinds. When paired with the precision of the fuel model, described below, the altitude optimization model finds the lowest cost path, the safest path, and the fastest path, presenting all as options for pilots to take advantage of. Additionally, speed settings are included in the recommendation to provide maximum control over fuel savings. Turbulence thresholds can be tailored as well, meaning that recommended paths will achieve the best efficiency available within a given passenger comfort and safety range.
4.6-4.9%
fuel reduction (average maximum) during cruise phase
Feature 02
Fuel model
In order to precisely avoid turbulence, high fidelity fuel consumption prediction is essential. By combining physical and machine learning models, we have successfully attained high precision fuel consumption predictions that account for the unique characteristics of each aircraft at the tail number level. This enables Untangle to accurately estimate fuel flow across any given trajectory. When utilized in Altitude Optimization, it accurately estimates the fuel consumption of the original flight plan, as well as the cost of all flyable segments, in order to find to lowest cost path, thereby generating an optimized route.
More information will be available in our next white paper.
Products
The features of Untangle are packaged as two products:
API
Untangle API provides for integration for existing EFBs, and other platforms to benefit from Untangle features.
EFB
Our EFB product, called Weave, enables users to access and visualize Untangle features from any device.
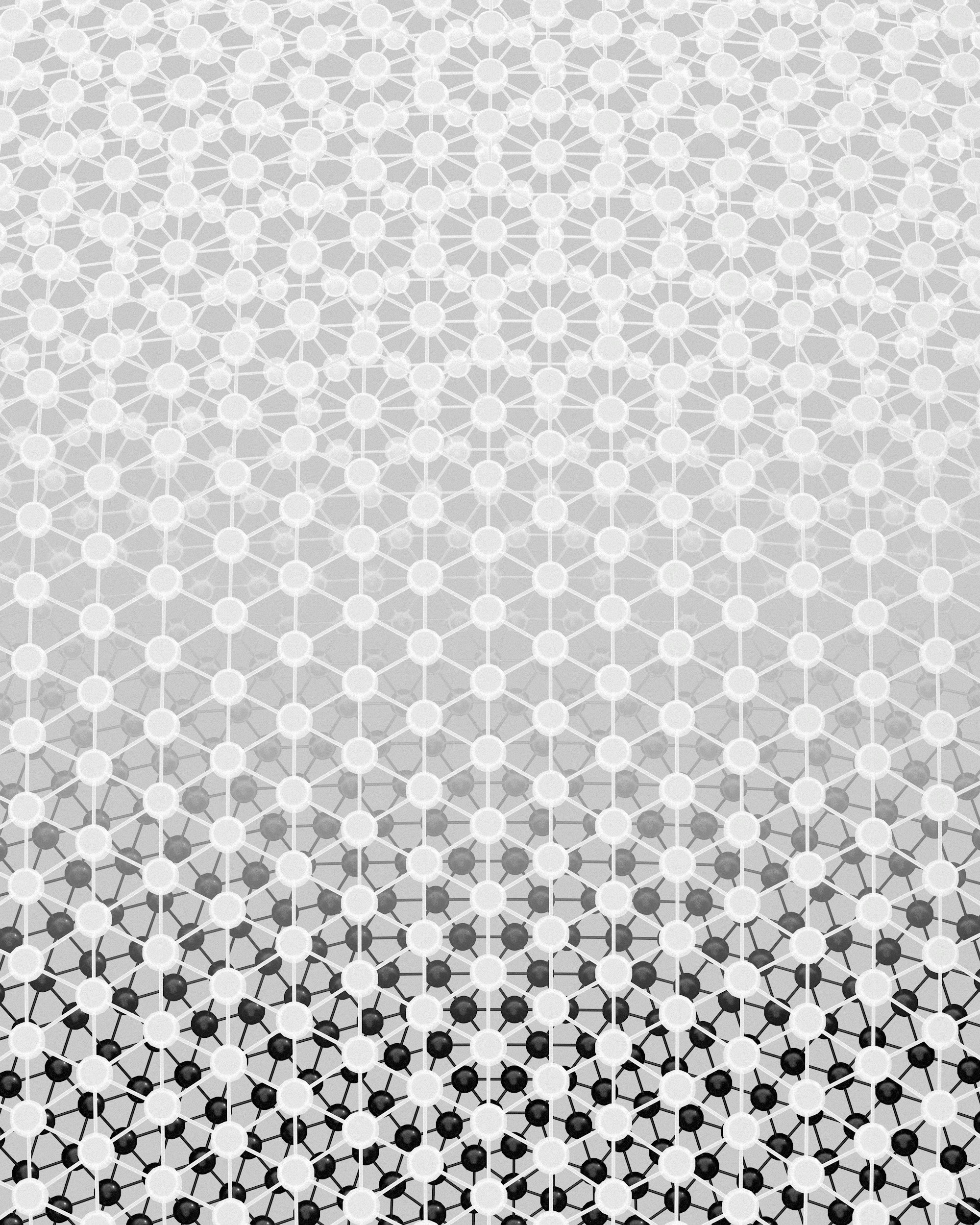
Our vision
We aim to unlock a new era of flight and achieve comprehensive efficiency by applying new ML/AI techniques, and building deeply coordinated solutions that will drive operational efficiency and decarbonization.
Collaborators and Partners
We are proud to collaborate with our fantastic academic, industry and airline partners:
Peach Aviation
The University of Tokyo
Department of Aeronautics and Astronautics, The University of Tokyo
JAXA
Development Bank of Japan
Aerospace Xelerated
The Boeing Company
Tawazun Council
Reach out
If your research, or commercial interests overlap, please feel free to get in touch to explore the possibility of collaboration.